ChatGPT Wrong Answers Examples: Common Mistakes and How It Affects AI Accuracy
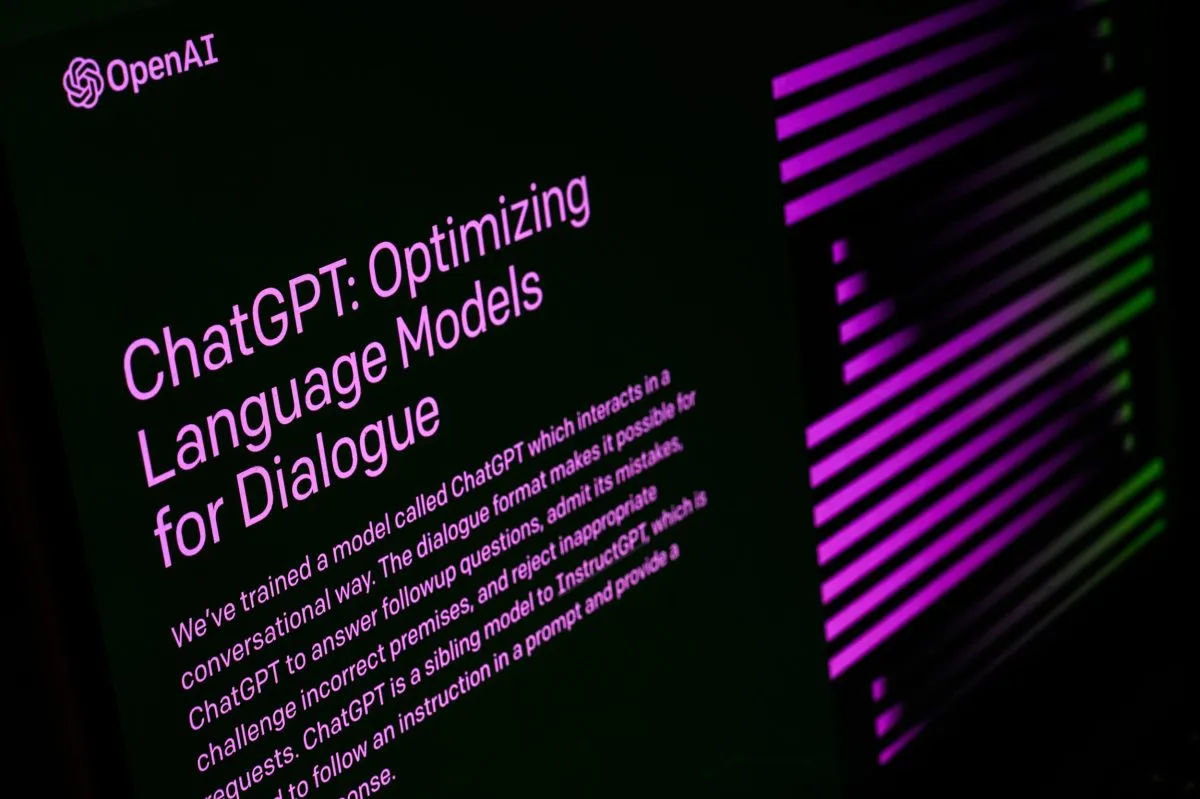
Artificial intelligence, particularly large language models (LLMs) like ChatGPT, has rapidly transformed various aspects of our lives, from content creation to customer service. However, despite its impressive capabilities, ChatGPT is not infallible. It can sometimes generate incorrect, misleading, or nonsensical answers, a phenomenon that raises concerns about its reliability and the broader implications for AI accuracy. Understanding the nature of these errors, their causes, and potential mitigation strategies is crucial for responsible AI development and deployment.
The Nature of ChatGPT's Errors
ChatGPT's errors manifest in various forms. One common type is factual inaccuracy. The model might present information that is simply wrong, citing nonexistent sources, misrepresenting historical events, or fabricating details. This can be particularly problematic when users rely on ChatGPT for research, education, or decision-making. Imagine a student using ChatGPT to write a history paper, only to unknowingly include fabricated historical events. The consequences could range from a failing grade to the spread of misinformation.
Another type of error is logical inconsistency. ChatGPT might generate responses that contradict themselves or violate basic principles of logic. This can occur when the model attempts to synthesize information from multiple sources or when it encounters ambiguous or contradictory prompts. For example, it might provide conflicting advice on a financial matter or offer solutions to a problem that are mutually exclusive. Such inconsistencies can undermine the user's trust in the model and make it difficult to rely on its output.
Furthermore, ChatGPT can sometimes produce nonsensical or irrelevant responses. This can happen when the model encounters prompts that are poorly worded, ambiguous, or outside its training data. In such cases, the model might generate text that is grammatically correct but lacks coherence or meaning. This can be frustrating for users who are seeking clear and concise answers to their questions. It also highlights the limitations of the model's understanding of the world and its ability to reason about complex concepts.
Finally, ChatGPT is susceptible to biases present in its training data. This means that the model might generate responses that reflect societal stereotypes, prejudices, or discriminatory attitudes. For example, it might perpetuate gender stereotypes in its descriptions of different professions or exhibit racial biases in its responses to questions about crime. These biases can have harmful consequences, reinforcing existing inequalities and perpetuating harmful stereotypes. Addressing these biases is a critical challenge for AI developers.
Underlying Causes of Inaccurate Responses
Several factors contribute to ChatGPT's tendency to generate inaccurate responses. One key factor is the nature of its training data. ChatGPT is trained on a massive dataset of text and code, but this dataset is not perfect. It may contain errors, biases, and inconsistencies that are reflected in the model's output. The model learns to identify patterns and relationships in the data, but it does not necessarily understand the underlying meaning or truthfulness of the information. As a result, it can sometimes generate responses that are statistically plausible but factually incorrect.
Another contributing factor is the model's reliance on statistical correlations rather than genuine understanding. ChatGPT does not understand the world in the same way that humans do. It does not have common sense, real-world experience, or the ability to reason about cause and effect. Instead, it relies on statistical patterns in its training data to generate responses. This means that it can sometimes make mistakes when it encounters situations that are outside its training data or that require more sophisticated reasoning.
The architecture of the model itself can also contribute to errors. ChatGPT is a complex neural network with millions of parameters. While this complexity allows it to generate impressive text, it also makes it difficult to understand how the model works and why it makes certain mistakes. The model's internal representations are often opaque and difficult to interpret, making it challenging to identify and correct the underlying causes of errors.
Furthermore, the way in which ChatGPT is prompted can influence the accuracy of its responses. Ambiguous, poorly worded, or leading prompts can confuse the model and lead to inaccurate or irrelevant answers. For example, if a user asks a question that is open to multiple interpretations, the model might choose the wrong interpretation and provide an incorrect answer. Similarly, if a user includes biased or misleading information in their prompt, the model might incorporate that information into its response, perpetuating the bias.
Impact on AI Accuracy and Reliability
The tendency of ChatGPT to generate inaccurate responses has significant implications for the accuracy and reliability of AI systems. It raises concerns about the trustworthiness of AI-generated content and the potential for misinformation to spread. If users cannot rely on ChatGPT to provide accurate information, they may be less likely to trust AI systems in general. This could hinder the adoption of AI technology and limit its potential benefits.
Inaccurate responses can also have practical consequences in various domains. In healthcare, for example, incorrect diagnoses or treatment recommendations could have serious implications for patient safety. In finance, inaccurate investment advice could lead to financial losses. In education, incorrect information could hinder student learning. It is therefore crucial to address the issue of accuracy in AI systems to ensure that they are used responsibly and effectively.
The presence of biases in ChatGPT's responses also raises ethical concerns. Biased AI systems can perpetuate societal inequalities and discriminate against certain groups of people. This can have harmful consequences for individuals and communities, reinforcing existing prejudices and limiting opportunities. Addressing these biases is essential for ensuring that AI systems are fair and equitable.
Moreover, the lack of transparency in ChatGPT's decision-making process makes it difficult to understand why it generates certain responses. This lack of transparency can undermine trust in the system and make it difficult to hold it accountable for its errors. Improving the transparency of AI systems is crucial for building trust and ensuring that they are used responsibly.
Strategies for Mitigating Errors and Improving Accuracy
Several strategies can be employed to mitigate errors and improve the accuracy of ChatGPT and similar LLMs. One approach is to improve the quality and diversity of the training data. This involves carefully curating the data to remove errors, biases, and inconsistencies. It also involves including a wider range of perspectives and experiences to ensure that the model is exposed to a more representative sample of the world.
Another strategy is to develop more sophisticated techniques for detecting and correcting errors in the model's output. This could involve using machine learning algorithms to identify potentially inaccurate or biased responses and flagging them for human review. It could also involve developing methods for automatically correcting errors in the text, such as using knowledge graphs or external databases to verify factual claims.
Improving the model's architecture and training process can also enhance accuracy. This could involve using more advanced neural network architectures that are better able to capture complex relationships in the data. It could also involve using different training techniques, such as reinforcement learning, to encourage the model to generate more accurate and reliable responses.
Furthermore, providing users with clear guidelines on how to prompt the model effectively can improve the quality of its responses. This could involve providing examples of good and bad prompts, as well as tips on how to avoid ambiguity and bias. It could also involve developing tools that automatically analyze prompts and provide feedback to users on how to improve them.
Finally, incorporating human oversight into the AI development and deployment process is crucial for ensuring accuracy and reliability. This could involve having human experts review the model's output, provide feedback on its performance, and identify potential biases or errors. It could also involve establishing clear lines of accountability for the model's actions, so that users know who to contact if they encounter problems.
The Future of AI Accuracy
The quest for greater accuracy in AI systems is an ongoing process. As AI technology continues to evolve, we can expect to see further improvements in the accuracy and reliability of LLMs like ChatGPT. However, it is important to recognize that AI systems are unlikely to ever be perfect. They will always be susceptible to errors, biases, and limitations. Therefore, it is crucial to approach AI technology with a critical and informed perspective, recognizing its potential benefits while also being aware of its potential risks.
The future of AI accuracy will depend on a combination of technical advancements, ethical considerations, and societal norms. We need to develop more sophisticated algorithms, address biases in training data, and establish clear guidelines for responsible AI development and deployment. We also need to foster a culture of transparency and accountability, so that users can trust AI systems and hold them accountable for their actions.
Ultimately, the goal is to create AI systems that are not only accurate but also beneficial to society. This requires a collaborative effort involving researchers, developers, policymakers, and the public. By working together, we can ensure that AI technology is used to solve real-world problems, improve people's lives, and promote a more just and equitable world.
The journey toward achieving truly reliable and accurate AI is a marathon, not a sprint. Continuous research, rigorous testing, and ethical considerations must be at the forefront of development. Only then can we harness the full potential of AI while mitigating the risks associated with inaccurate or biased outputs.
✦ Tanya AI