ChatGPT Hallucination Explained: Why It Happens and Its Impact on AI Reliability
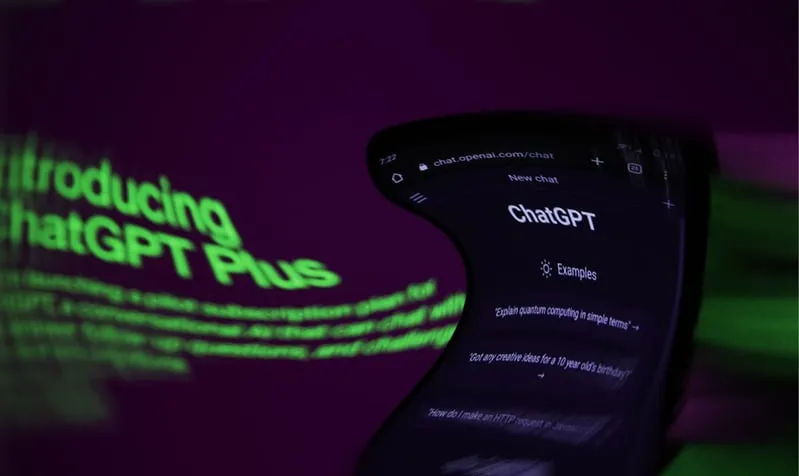
Large Language Models (LLMs) like ChatGPT have revolutionized the way we interact with artificial intelligence. Their ability to generate human-quality text, translate languages, and answer complex questions has made them invaluable tools in various fields. However, a significant challenge remains: the phenomenon of hallucination, where these models confidently produce incorrect, nonsensical, or even fabricated information. Understanding why these hallucinations occur and their impact on the reliability of AI is crucial for responsible development and deployment of LLMs.
What are ChatGPT Hallucinations?
ChatGPT hallucinations, in the context of AI, refer to instances where the model generates outputs that are factually incorrect, misleading, or completely made up. These aren't simply errors in calculation or minor inaccuracies; they are instances where the model presents false information as if it were true, often with a high degree of confidence. This can manifest in various ways, including inventing sources, fabricating events, or providing incorrect definitions. The term hallucination is used metaphorically, drawing a parallel to human hallucinations where individuals perceive things that are not actually present.
It's important to distinguish between different types of errors. A simple factual error, like stating the wrong date for a historical event, might be considered a mistake. However, a hallucination goes beyond this. It might involve creating an entirely fictional historical event, attributing it to a non-existent source, and presenting it as a verifiable fact. The key characteristic is the model's confident presentation of false information as truth.
The consequences of these hallucinations can be significant. In applications where accuracy is paramount, such as medical diagnosis or legal research, relying on hallucinated information could lead to serious errors with real-world consequences. Furthermore, the spread of misinformation through AI-generated content can erode trust in these technologies and contribute to the broader problem of fake news.
Why Do Hallucinations Occur?
The reasons behind ChatGPT hallucinations are complex and multifaceted, stemming from the architecture of the models, the nature of the training data, and the inherent limitations of statistical language modeling. Several key factors contribute to this phenomenon:
1. Training Data Imperfections: LLMs are trained on massive datasets scraped from the internet. While these datasets are vast, they are not perfect. They can contain inaccuracies, biases, and contradictory information. The model learns to identify patterns and relationships within this data, but it also inadvertently absorbs the errors and biases present. If the training data contains misinformation about a particular topic, the model is likely to reproduce that misinformation in its output.
Furthermore, the distribution of information within the training data can influence the model's behavior. If certain facts or perspectives are overrepresented, the model may be more likely to generate outputs that reflect those biases, even if they are not entirely accurate. The sheer scale of the training data makes it difficult to curate and verify every piece of information, making it almost inevitable that some inaccuracies will slip through.
2. Statistical Language Modeling: LLMs are fundamentally statistical models. They predict the next word in a sequence based on the preceding words and the patterns they have learned from the training data. They don't understand the meaning of the words in the same way that humans do. Instead, they rely on statistical correlations to generate text. This means that the model can generate grammatically correct and seemingly coherent text without necessarily understanding the underlying facts or logic.
This statistical approach can lead to hallucinations when the model encounters a situation where it lacks sufficient information or when the patterns in the training data are ambiguous. In these cases, the model may rely on its learned associations to generate a plausible but ultimately incorrect response. The model is essentially guessing based on the available evidence, and sometimes those guesses are wrong.
3. Over-Reliance on Patterns: LLMs are adept at identifying and replicating patterns in the training data. This is what allows them to generate human-quality text. However, this ability can also be a source of hallucinations. The model may identify a pattern that is generally true but does not apply in a specific context. It may then apply that pattern inappropriately, leading to an incorrect or nonsensical output.
For example, if the training data contains many examples of people being described as experts in a particular field, the model may be more likely to label someone as an expert even if there is no evidence to support that claim. This over-reliance on patterns can lead to the creation of fictional experts or the misattribution of expertise.
4. Lack of Real-World Understanding: LLMs operate solely on textual data. They do not have direct access to the real world or the ability to experience things firsthand. This lack of real-world understanding can make it difficult for them to distinguish between fact and fiction. They may be able to generate text that sounds plausible, but they lack the common sense and background knowledge that humans use to evaluate the truthfulness of information.
For instance, if asked to describe the process of baking a cake, the model might be able to generate a detailed recipe. However, it would not understand the physical properties of the ingredients or the practical challenges involved in baking. This lack of real-world grounding can make it more susceptible to generating nonsensical or impossible scenarios.
5. The Black Box Problem: The internal workings of LLMs are often opaque, even to the researchers who develop them. These models are complex neural networks with millions or even billions of parameters. It can be difficult to understand exactly why a model generates a particular output. This black box nature of LLMs makes it challenging to diagnose and correct the causes of hallucinations. Researchers are constantly working on techniques to improve the interpretability of these models, but it remains a significant challenge.
The Impact on AI Reliability
Hallucinations pose a significant threat to the reliability of AI systems. If users cannot trust the information generated by these models, they will be less likely to use them, especially in critical applications. The impact of hallucinations can be felt across various domains:
1. Erosion of Trust: The most immediate impact of hallucinations is the erosion of trust in AI systems. If a user encounters a hallucination, they may become skeptical of the model's overall accuracy and reliability. This can lead to a reluctance to use the model for important tasks or to rely on its output without independent verification. Building and maintaining trust is crucial for the widespread adoption of AI, and hallucinations undermine this process.
2. Misinformation and Disinformation: LLMs have the potential to generate and spread misinformation on a massive scale. Hallucinations can contribute to the creation of fake news articles, fabricated scientific studies, and other forms of misleading content. This can have serious consequences for public discourse, political decision-making, and social cohesion. The ability of AI to generate realistic and persuasive text makes it a powerful tool for spreading disinformation.
3. Legal and Ethical Concerns: The use of LLMs in sensitive areas such as legal advice, medical diagnosis, and financial planning raises significant legal and ethical concerns. If a model hallucinates information that leads to a wrong decision, who is responsible? Is it the developer of the model, the user who relied on the information, or someone else? These questions are complex and require careful consideration. The potential for harm caused by hallucinations necessitates the development of clear legal and ethical guidelines for the use of LLMs.
4. Impaired Decision-Making: In business and other professional settings, relying on hallucinated information can lead to poor decision-making. For example, if a marketing team uses an LLM to generate market research data and the model hallucinates key findings, the team may make incorrect assumptions about customer preferences and develop ineffective marketing campaigns. The cost of these errors can be significant, both in terms of financial losses and reputational damage.
5. Hindered Innovation: The presence of hallucinations can also hinder innovation in AI. If researchers and developers are constantly struggling to overcome the problem of hallucinations, they may be less likely to explore new and innovative applications of LLMs. The focus shifts from pushing the boundaries of what is possible to simply trying to make the models more reliable. This can slow down the overall progress of AI research and development.
Mitigation Strategies
Addressing the problem of ChatGPT hallucinations requires a multi-pronged approach that involves improving the training data, refining the model architecture, and developing new techniques for detecting and mitigating hallucinations. Several strategies are being actively pursued:
1. Data Curation and Augmentation: Improving the quality and diversity of the training data is crucial. This involves carefully curating the data to remove inaccuracies, biases, and contradictory information. It also involves augmenting the data with additional examples to improve the model's understanding of different topics and contexts. Techniques such as data synthesis and data augmentation can be used to create new training examples that are more representative of the real world.
2. Fact Verification and Knowledge Integration: Integrating external knowledge sources into LLMs can help to reduce hallucinations. This involves providing the model with access to factual databases, knowledge graphs, and other sources of verified information. The model can then use this external knowledge to check the accuracy of its own outputs and to correct any errors. Techniques such as retrieval-augmented generation (RAG) are being used to incorporate external knowledge into the generation process.
3. Model Architecture Improvements: Researchers are exploring new model architectures that are less prone to hallucinations. This includes techniques such as attention mechanisms, which allow the model to focus on the most relevant parts of the input data, and memory networks, which allow the model to store and retrieve information from past interactions. These architectural improvements can help the model to generate more accurate and consistent outputs.
4. Hallucination Detection Techniques: Developing methods for detecting hallucinations is essential for preventing the spread of misinformation. This involves training models to identify outputs that are likely to be false or misleading. Techniques such as uncertainty estimation and adversarial training can be used to improve the accuracy of hallucination detection. These detection methods can be used to flag potentially problematic outputs and to alert users to the possibility of errors.
5. Human-in-the-Loop Approaches: Incorporating human feedback into the training and deployment of LLMs can help to reduce hallucinations. This involves having human experts review the model's outputs and provide feedback on their accuracy and relevance. This feedback can then be used to fine-tune the model and to improve its performance. Human-in-the-loop approaches can also be used to monitor the model's behavior in real-world settings and to identify any potential problems.
The Future of AI Reliability
Addressing the challenge of ChatGPT hallucinations is essential for ensuring the reliability and trustworthiness of AI systems. While significant progress has been made in recent years, much work remains to be done. The future of AI reliability depends on continued research and development in areas such as data curation, model architecture, and hallucination detection. It also requires a collaborative effort between researchers, developers, policymakers, and the public to establish clear ethical guidelines and best practices for the use of LLMs.
As AI technology continues to evolve, it is crucial to prioritize accuracy and reliability. This means investing in the development of robust and trustworthy AI systems that can be used safely and responsibly. By addressing the problem of hallucinations and other challenges, we can unlock the full potential of AI to benefit society.
Ultimately, the goal is to create AI systems that are not only intelligent but also reliable, transparent, and accountable. This requires a commitment to ethical principles and a focus on building AI that serves the best interests of humanity.
✦ Tanya AI